Disruptive technologies in the Iberian ham industry to ensure food safety
SPECTROMEAT arises in response to the growing demand for efficient, non-destructive and fast methods for quality control, especially in the meat sector. The main objective of this project is the development of a sensor based on NIR and/or fluorescence spectroscopy for real-time monitoring of the presence of Listeria during the maturation process of cured sausages.
This technological advance not only strengthens the competitiveness of companies in the sector (particularly SMEs), but also guarantees the food safety of their products.
The consortium behind SPECTROMEAT includes COVAP as representative of the meat industry, Nulab focusing on portable NIR technologies, ASINCAR that will validate the tested technologies, Radiantis with technological support, and UAB exploring fluorescence for pathogen detection. Coordination between the entities has been carried out by secpho, Deep Tech cluster.
The initiative has received funding from the Ministry of Industry, Trade and Tourism corresponding to Next Generation EU funds, canalised through the support programme for Innovative Business Groups, whose objective is to digitalise industry.
In a context of stringent regulations, such as those in the U.S. with “Listeria 0”, rapid pathogen detection becomes essential.
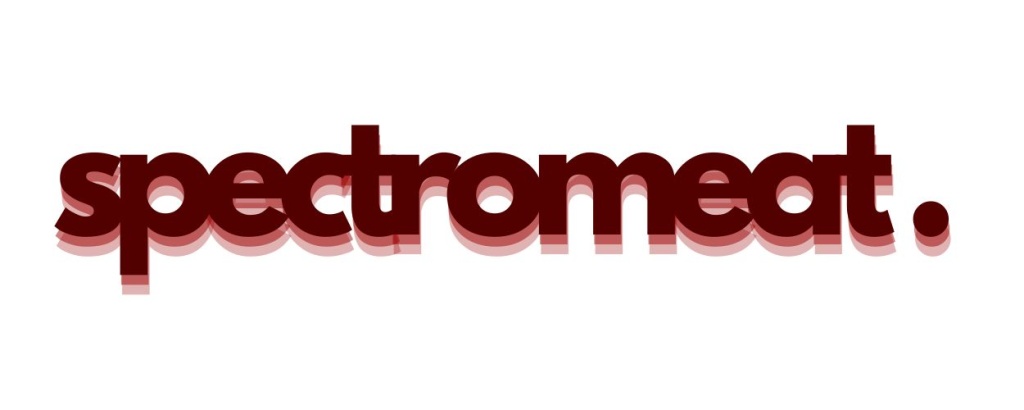
The increasing penetration of digitization and artificial intelligence-based strategies in the food industry has generated an ever more pressing demand for fast, inexpensive, non-destructive, reliable, reproducible and robust methods for raw material, process and quality control.
For the meat industry, especially that of cured Iberian cured meats, it is vital to obtain physical-chemical and microbiological information in real time in order to ensure food quality and safety. Control during the drying/curing process, crucial for the production of cured meats, becomes an economic and safety challenge.
With this need, the SPECTROMEAT project was born, which seeks to develop a sensor based on emerging technologies, such as NIR and fluorescence spectroscopy, to measure water and moisture activity in sausages non-destructively and in real time to detect Listeria.
The application of AI will simplify the development of predictive models and facilitate decision making based on real-time measurements.
This non-destructive approach offers several advantages, such as the ability to measure accurately without sacrificing samples, production efficiency, reduced waste and adjustment time in the drying and ripening process. In addition, real-time detection of Listeria events would enable a rapid response to stop the spread and prevent contamination of the final product, meeting the highest food safety standards.
Near infrared spectroscopy (NIR) is proposed as an effective technology for measuring major food components, but its adoption by SMEs is limited by the cost of the equipment. SPECTROMEAT seeks to overcome this obstacle by developing small, lower cost equipment tailored to the needs of the food industry, especially SMEs. The application of these emerging technologies would not only provide new quality control and food safety parameters, but would also represent a competitive advantage to open new opportunities in demanding international food safety markets.
Regarding technological development, the proposal includes the research and development of a portable NIR technology for the control of physicochemical parameters, as well as the exploration of fluorescence for the detection of Listeria in meat products. The resulting infrastructure will enable the connectivity of portable equipment to a digital cloud platform, facilitating the integration of predictive models based on artificial intelligence. This would overcome the main limitation of NIR technology, which is massive data generation and the need for specialists in NIRS and chemometric techniques.
Ultimately, the SPECTROMEAT project represents a significant advance in the application of emerging photonic techniques for quality control and food safety in the meat industry. The results and predictive models generated will serve as a starting point for future expansions to other products and processes, marking a very broad expected scope and contributing to the digital transformation of the agri-food sector.